SDPoint is a concept that is gaining traction in various industries, particularly in data science, technology, and business analytics. It refers to a specific metric or scoring system used to evaluate performance, efficiency, or quality. This article will delve into the intricacies of SDPoint, its applications, and its significance in modern industries.
As businesses and organizations increasingly rely on data-driven decision-making, understanding concepts like SDPoint becomes crucial. Whether you're a data scientist, a business analyst, or a tech enthusiast, this article will provide valuable insights into the world of SDPoint and its applications.
In this guide, we will explore the origins of SDPoint, its practical uses, and how it can be leveraged to enhance performance and efficiency. By the end of this article, you will have a comprehensive understanding of SDPoint and its relevance in today's data-centric world.
Read also:Kevin Selleck A Rising Star In Hollywood
Table of Contents
- Introduction to SDPoint
- History of SDPoint
- Key Components of SDPoint
- Applications of SDPoint
- Advantages and Challenges
- SDPoint in Data Science
- SDPoint in Business Analytics
- How to Calculate SDPoint
- Case Studies
- Future of SDPoint
Introduction to SDPoint
SDPoint, or Standard Deviation Point, is a statistical metric used to measure variability or dispersion in a dataset. It is widely used in data science, finance, and business analytics to assess risk, performance, and efficiency. Understanding SDPoint is essential for professionals who rely on data to make informed decisions.
Why SDPoint Matters
SDPoint provides a quantitative measure of how much individual data points deviate from the mean. This metric is particularly useful in identifying outliers, assessing data quality, and making predictions. By incorporating SDPoint into their workflows, organizations can gain deeper insights into their data and improve decision-making processes.
History of SDPoint
The concept of SDPoint has its roots in statistical analysis and probability theory. It was first introduced by statisticians who sought to develop a standardized way to measure variability in datasets. Over time, SDPoint has evolved to become an essential tool in various fields, including finance, engineering, and healthcare.
Key Milestones in the Development of SDPoint
- Early 20th century: Introduction of standard deviation as a statistical measure.
- 1950s: Application of standard deviation in quality control processes.
- 1980s: Integration of SDPoint into financial risk management models.
- 2000s: Adoption of SDPoint in data science and machine learning algorithms.
Key Components of SDPoint
SDPoint is composed of several key elements that contribute to its effectiveness as a statistical tool. These components include:
- Mean: The average value of a dataset.
- Variance: The average of the squared differences from the mean.
- Standard Deviation: The square root of variance, representing the spread of data points.
- Data Points: Individual values in a dataset used to calculate SDPoint.
How These Components Work Together
By combining these elements, SDPoint provides a comprehensive view of data variability. This allows analysts to identify patterns, detect anomalies, and make informed predictions.
Applications of SDPoint
SDPoint has numerous applications across various industries. Some of the most common uses include:
Read also:Unveiling The Lifespan Of Marigolds Are Marigolds Annual Or Perennial
- Finance: Assessing investment risk and portfolio performance.
- Healthcare: Evaluating patient outcomes and treatment efficacy.
- Manufacturing: Monitoring production quality and process efficiency.
- Marketing: Analyzing customer behavior and campaign effectiveness.
Real-World Examples
For instance, in finance, SDPoint is used to calculate the volatility of stock prices, helping investors make informed decisions. In healthcare, it is employed to measure the consistency of treatment results, ensuring optimal patient care.
Advantages and Challenges
While SDPoint offers numerous benefits, it also presents some challenges. Understanding these aspects is crucial for effective implementation.
Advantages of SDPoint
- Provides a standardized measure of variability.
- Helps identify outliers and anomalies in datasets.
- Supports data-driven decision-making processes.
Challenges of SDPoint
- Requires a solid understanding of statistical concepts.
- May not be suitable for all types of datasets.
- Can be computationally intensive for large datasets.
SDPoint in Data Science
Data science relies heavily on statistical tools like SDPoint to extract meaningful insights from complex datasets. By leveraging SDPoint, data scientists can uncover patterns, detect trends, and make accurate predictions.
Use Cases in Data Science
Some common use cases of SDPoint in data science include:
- Anomaly detection in sensor data.
- Clustering analysis for customer segmentation.
- Feature selection in machine learning models.
SDPoint in Business Analytics
In business analytics, SDPoint plays a vital role in evaluating performance metrics and optimizing operational efficiency. Organizations use SDPoint to assess key performance indicators (KPIs) and identify areas for improvement.
Applications in Business Analytics
- Measuring sales performance across different regions.
- Monitoring customer satisfaction scores.
- Assessing supply chain efficiency and inventory management.
How to Calculate SDPoint
Calculating SDPoint involves several steps, including determining the mean, variance, and standard deviation of a dataset. Below is a step-by-step guide:
- Calculate the mean of the dataset.
- Find the squared difference between each data point and the mean.
- Compute the average of these squared differences (variance).
- Take the square root of the variance to obtain the standard deviation.
Tools for Calculating SDPoint
Several tools and software are available to simplify the calculation of SDPoint, including Excel, Python, and R. These tools offer built-in functions and libraries to streamline the process.
Case Studies
To illustrate the practical applications of SDPoint, let's explore a few real-world case studies:
Case Study 1: Financial Risk Management
A global investment firm used SDPoint to assess the risk associated with its investment portfolio. By analyzing historical stock price data, the firm was able to identify high-risk assets and adjust its investment strategy accordingly.
Case Study 2: Healthcare Quality Improvement
A hospital implemented SDPoint to evaluate the consistency of patient outcomes across different departments. The results helped identify areas for improvement and led to enhanced patient care.
Future of SDPoint
As technology continues to advance, the role of SDPoint in data analysis is expected to grow. Emerging trends such as artificial intelligence and big data analytics will further enhance the capabilities of SDPoint, making it an indispensable tool for professionals in various fields.
Potential Developments
- Integration with AI-driven analytics platforms.
- Expansion into new industries and applications.
- Enhanced computational efficiency for large-scale datasets.
Conclusion
SDPoint is a powerful statistical metric that provides valuable insights into data variability and performance. Its applications span across multiple industries, making it an essential tool for data scientists, analysts, and professionals alike. By understanding and leveraging SDPoint, organizations can enhance their decision-making processes and achieve better outcomes.
We encourage you to explore the resources mentioned in this article and apply SDPoint to your own datasets. Don't forget to leave a comment or share this article with your network. For more informative content, check out our other articles on data science and analytics.

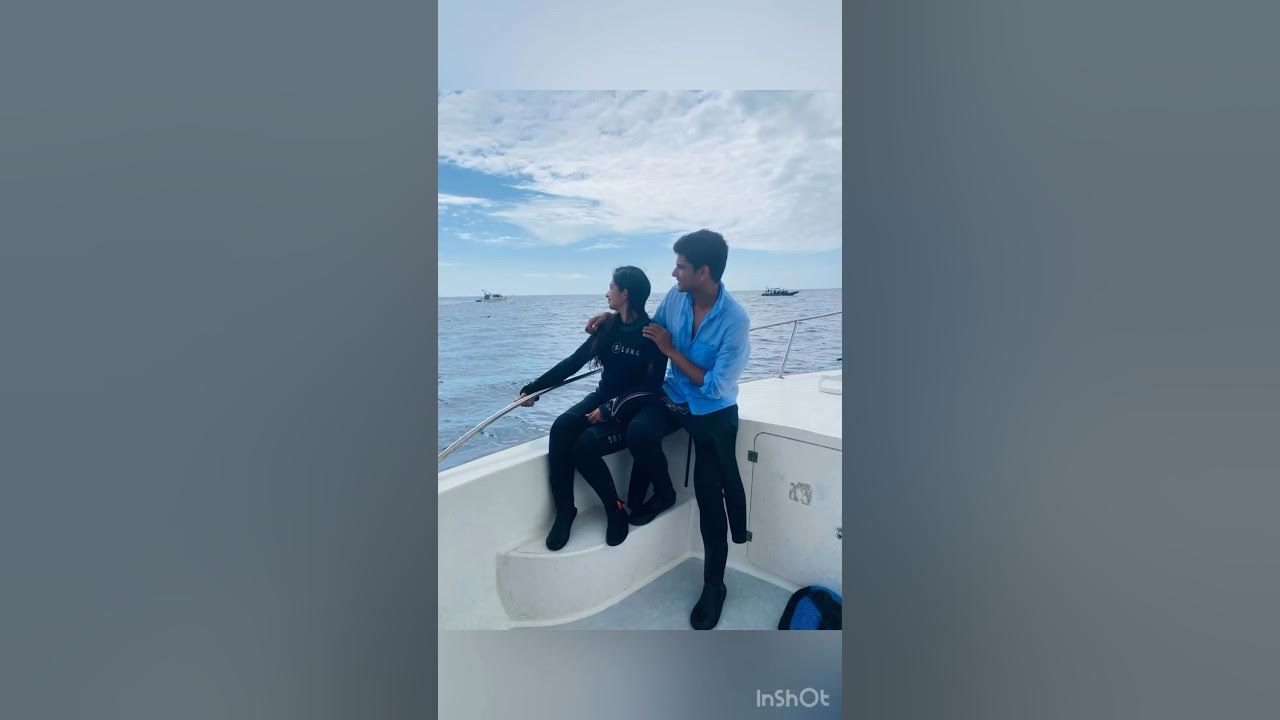